MonoPatchNeRF: Improving Neural Radiance Fields with Patch-based Monocular Guidance
- Yuqun Wu1*
- Jae Yong Lee1*
- Chuhang Zou2
- Shenlong Wang1
- Derek Hoiem1
- 1University of Illinois at Urbana-Champaign
- 2Amazon
- * Equal Contribution
Abstract
The latest regularized Neural Radiance Field (NeRF) approaches produce poor geometry and view extrapolation for multiview stereo (MVS) benchmarks such as ETH3D. In this paper, we aim to create 3D models that provide accurate geometry and view synthesis, partially closing the large geometric performance gap between NeRF and traditional MVS methods. We propose a patch-based approach that effectively leverages monocular surface normal and relative depth predictions. The patch-based ray sampling also enables the appearance regularization of normalized cross-correlation (NCC) and structural similarity (SSIM) between randomly sampled virtual and training views. We further show that “density restrictions” based on sparse structure-from-motion points can help greatly improve geometric accuracy with a slight drop in novel view synthesis metrics. Our experiments show 4x the performance of RegNeRF and 8x that of FreeNeRF on average F1@2cm for ETH3D MVS benchmark, suggesting a fruitful research direction to improve the geometric accuracy of NeRF-based models, and sheds light on a potential future approach to enable NeRF-based optimization to eventually outperform traditional MVS.
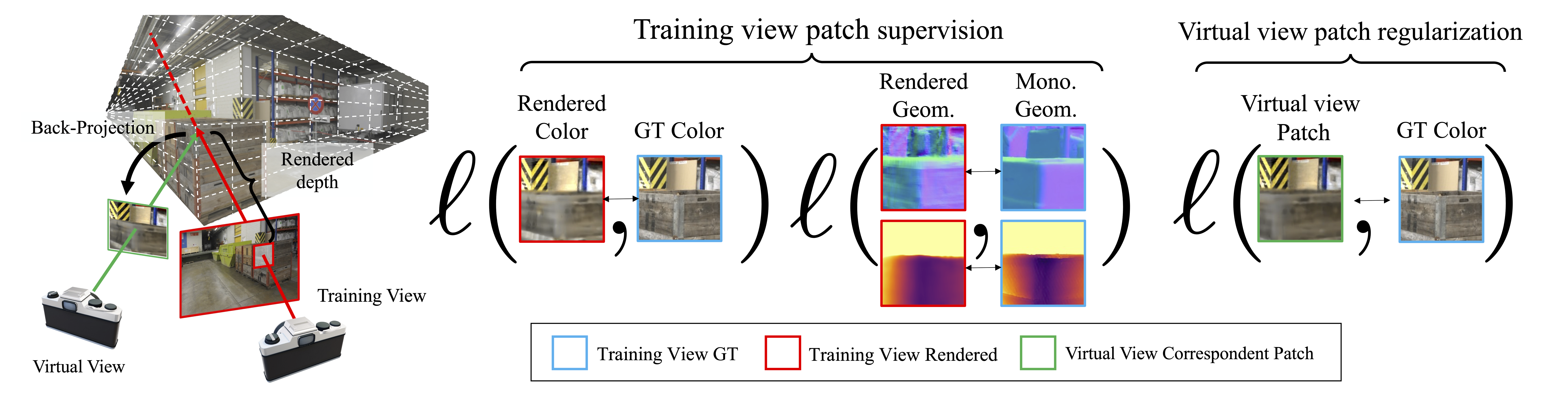
Free View Rendering
We provide video comparison between our method and three baselines(MonoSDF, RegNeRF, Neuralangelo) on ETH3D. Presented scenes are relief_2, facade, and kicker, with 31, 76, and 31 input views.
MonoSDF
RegNeRF
Neuralangelo
Acknowledgements
This work is supported in part by NSF IIS grants 2312102 and 2020227. SW is supported by NSF 2331878 and 2340254, and research grants from Intel, Amazon, and IBM. Thanks to Zhi-Hao Lin for sharing the code for pose interpolation and video generation.
The website template was borrowed from Michaël Gharbi, Ref-NeRF, Zip-NeRF, and ClimateNeRF.